F5 Labs Joins the Exploit Prediction Scoring System as a Data Partner | F5 Labs
by nlqip
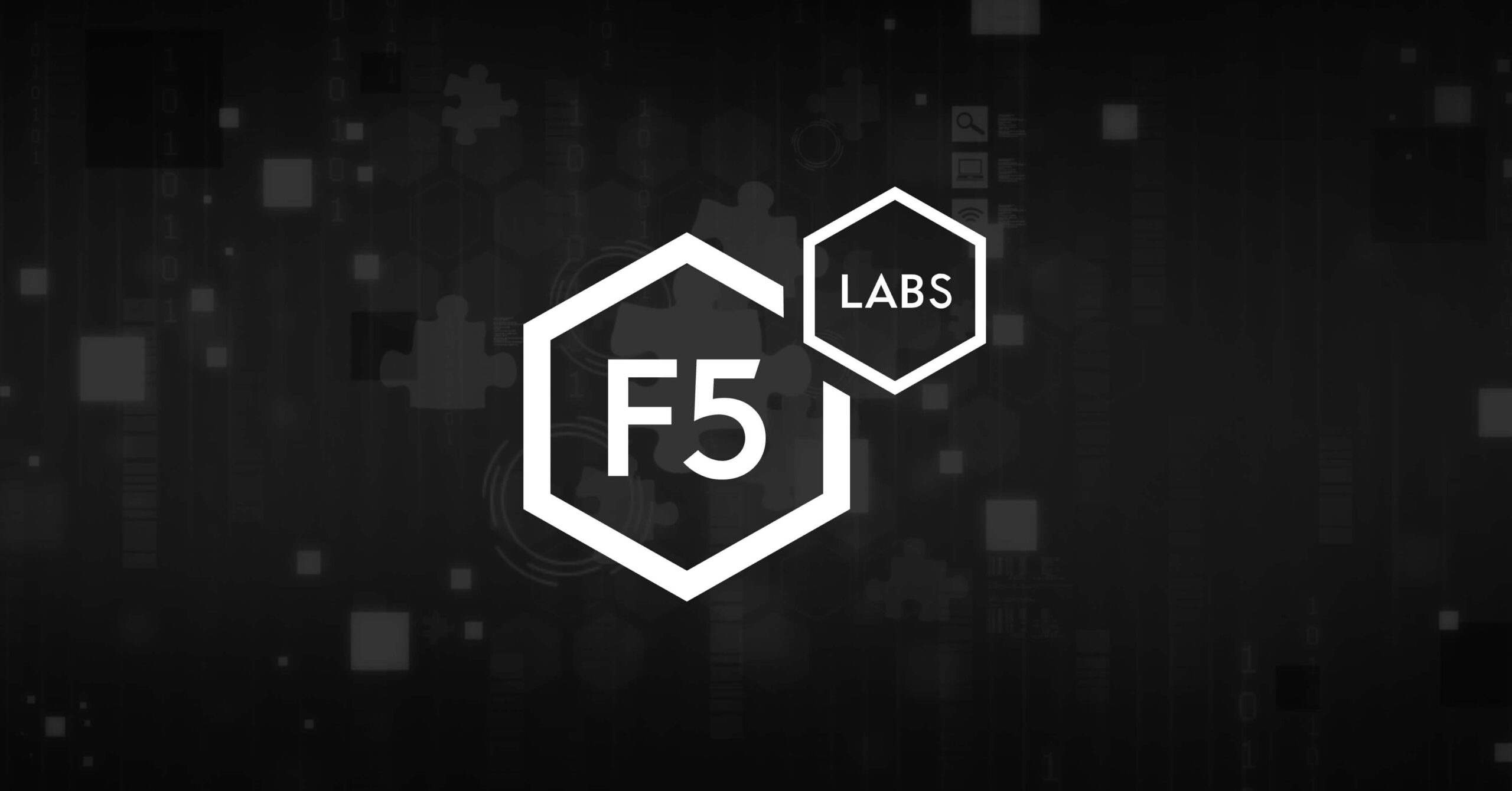
We are excited to announce that F5 Labs has become a data partner of the Exploit Prediction Scoring System (EPSS). The Internet-wide scanning and attempted exploitation activity that makes up our Sensor Intel Series also happens to be good training data for the machine learning system under EPSS’ hood.
F5 Labs wrote about EPSS in early 2020, a few months after it was unveiled at Black Hat 2019. In that article, we explored the vulnerability characteristics that the system was collecting for analysis at runtime, and used a specific example and the weightings for the most significant parameters in the model to calculate an EPSS score by hand.
Since then, however, EPSS has grown in prominence and adoption, the ML model is on its third iteration, and the performance is improved. We recently shipped our first batch of CVE-focused attack data to the EPSS team, so now seems like a good time to review what EPSS is, why we think it’s important, and how we fit into the picture.
What Is EPSS and What Problem Is It Solving?
The Exploit Prediction Scoring System is designed to help security teams prioritize vulnerability mitigation by calculating the 30-day likelihood that any given vulnerability will be exploited by an attacker. The need for this stems from two observations that security researchers have made in the last decade: the first is the observation that there are simply too many vulnerabilities in enterprise tech footprints to patch them all. A joint study by Kenna and Cyentia in 2019 found that across all enterprise sizes, organizations tend to mitigate about 10% of the open vulnerabilities in their systems.
The other observation is that a surprisingly small subset of vulnerabilities is exploited in the wild by attackers—around 5-6%. This means that patching 100% of open vulnerabilities is not only impossible in the long term, it is unnecessary. Patching all vulnerabilities would result in a lot of arguably unnecessary work since ~95% of those vulnerabilities represent minimal risk.
The problem, therefore, is to come up with a system to triage vulnerabilities so that we can use our limited remediation time to greatest effect. In theory the Common Vulnerability Scoring System (CVSS) should suffice, but this system has some limitations, most of which stem from the way that people use and interpret the scores. EPSS was specifically designed to outperform CVSS at the problem of ongoing vulnerability triage, and it does this through machine learning.
EPSS’ Machine Learning Model
At their most abstract, all machine learning systems require two kinds of inputs: training inputs and runtime inputs. The training inputs are used to determine optimum parameter weights for the model, and the runtime inputs are what the model then processes to produce its output. For EPSS the runtime inputs are a collection of roughly 1,500 vulnerability characteristics that are collected and updated daily. These are variables such as the existence of publicly available exploit code, the vendor of the affected system, and the technical impact of the vulnerability (such as remote code execution or directory traversal). Over the course of training the model will determine the relative importance of each of these variables to each vulnerability.
The daily update of these variables is one of the things that makes EPSS so useful. While CVSS has a temporal dimension, many organizations omit it and use CVSS as a static score. Since the true likelihood of exploitation is dynamic and ebbs and flows as these variables change, so does the EPSS score, which is why it is recalculated daily.
In addition to the runtime data, the ML system requires training data that it can use to determine the parameter weights. For this it needs many examples of attacker exploits in the wild. F5 Labs has just started contributing our own observations (more on this below) but EPSS also collects exploitation data from Cisco, AlienVault, Fortinet, GreyNoise, and others. Since this is a supervised learning model, these exploit attempts serve as annotations or data labels that provide feedback to the model as it determines weights. Perhaps the best way to understand this is to take an example and work backwards. If the “code execution” parameter is one of the most highly weighted in the EPSS system (Figure 1), we can reasonably infer that this characteristic showed up more often among the exploited CVEs in the training data irrespective of other characteristics like affected platform or natural language descriptions.
Source link
lol
We are excited to announce that F5 Labs has become a data partner of the Exploit Prediction Scoring System (EPSS). The Internet-wide scanning and attempted exploitation activity that makes up our Sensor Intel Series also happens to be good training data for the machine learning system under EPSS’ hood. F5 Labs wrote about EPSS in…
Recent Posts
- CISA Director Jen Easterly Stands Watch in the Cyberwars
- New Android Banking Malware ‘ToxicPanda’ Targets Users with Fraudulent Money Transfers
- Leveraging Wazuh for Zero Trust security
- Synology Urges Patch for Critical Zero-Click RCE Flaw Affecting Millions of NAS Devices
- Hackers Strike at Heart of Italian Government