Databricks CEO Ghodsi: Systems Integrator Partners Are Key To Winning ‘The AI Revolution’
by nlqip
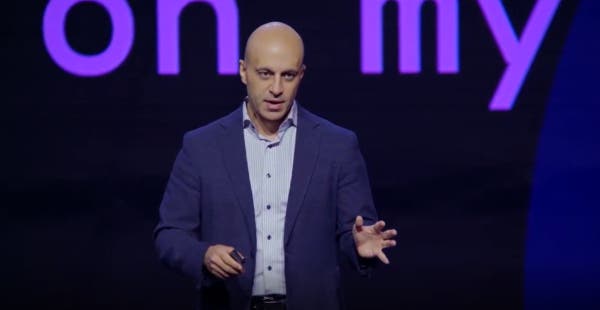
‘We both need to succeed together for Databricks to succeed,’ says Databricks co-founder and CEO Ali Ghodsi at the company’s Data+AI Summit.
Databricks is “doubling down” on working with its systems integrator partners, with co-founder and CEO Ali Ghodsi viewing services partners as an important part of the vendor’s go-to-market and widespread adoption of artificial intelligence tools.
Ghodsi spoke about the importance of systems integrators during a question-and-answer session at the San Francisco-based vendor’s Data+AI Summit.
“The AI revolution is not going to happen without the SIs,” Ghodsi said. “We both need to succeed together for Databricks to succeed.”
[RELATED: Databricks Data+AI Summit 2024: The Biggest News]
Databricks Data+AI Summit 2024
Databricks has more than 3,800 partners worldwide, according to the vendor.
Ghodsi said that the company is investing in more regional employees to help systems integrators in Asia, Europe and elsewhere. “We have to help them sell their services,” he said. “I’m connected to all the CEOs of the big GSIs [global systems integrators]. I meet them regularly. … Databricks would not be what it is without the GSIs and RSIs. And it’s going to be key to our success going forward as well.”
During the Databricks event, Ghodsi revealed new advancements in Databricks’ products and offered ways it seeks to set itself apart from the competition, particularly with data analytics rival Snowflake, which held its own conference in the same San Francisco conference center earlier in June.
Here’s more of what Ghodsi had to say during the Summit in his keynote and remarks to reporters.
Preventing Vendor Lock-In
We did a survey on our customers. And 85 percent of the [GenAI] use cases have not yet made it into production. … They’re still trying them out. … Every call I get on, people talk about fragmentation of the data estate … ‘We have so many different pieces of software. I don’t even know what they do. … We have to cut it down. I’m under budget pressure.’ … The consequence of this is lots of complexity, huge costs and then lock-in to these proprietary different systems.
Each of those systems is a little silo that you lock yourself into. … Stop giving your data to any vendors, OK? It doesn’t matter if it’s a proprietary data warehouse in the cloud or if it’s Snowflake or if it’s even Databricks. …
You should instead own your own data. … You should store it. … Pay for it independently. Make sure it has separated computer storage completely from the compute so it’s just a basic data lake. … That’s why we announced our open-source Delta Lake project here … a bunch of years ago. The idea was that suddenly we have this USB format.
And once we have this USB format, anyone can just plug in their data platform. Any of those vendors that I said don’t give your data to, they should just plug in your USB stick into that data that you have in the cloud, and then let the best engine win. … And also, it lets you get many more use cases because you can use different engines for different purposes if you want.
So this is our vision. Unfortunately, what happened is we almost succeeded. … There are now two camps. At Databricks, we have Delta Lake. We are seeing actually 92 percent of all of our data go to Delta. That’s about 4 exabytes of data every day. So 4,000 petabytes every day that’s processed going to Delta.
But there are also other vendors that are using this Apache Iceberg format. … Tabular [which Databricks acquired earlier this month] was founded by the original creators of the Apache Iceberg project. … The reason we did this is that we want this problem to go away so that you don’t have to pick which of the two silos, which of the USB formats do I have to store this in. … Whatever you store it in, all of the cables should just work.
So our strategy is, a year ago, here, we announced Project UniForm. … We’re actually announcing it in GA [general availability]. … We really, really want to double down on making sure that UniForm has full 100 percent compatibility and interoperability for both of us. … Then in the background, what we want to do is really work with these communities, the Delta Lake community and the Apache Iceberg community.
These are open-source communities. … We want to work with them and actually change the formats and bring them closer and closer to each other so that the differences between them do not matter.
You store your data in UniForm right now. Then over the next period of time, as the formats get closer and closer, it won’t even matter which one you have. The distinction will go away. And I hope within a year or two, we won’t even care.
Democratizing Data And AI
We want to democratize data. And second, we want to democratize AI. … Today, that is not true. Your CEO is not going to go access the data and ask questions from the data.
He or she will go to the data team and ask them, ‘Hey, can you give me this report?’ … because your CEO does not speak SQL or Python, or at least doesn’t know where to find the data. … So we’re really hoping that we can democratize this so if you speak English or any other natural language, you should just be able to ask your question for the data.
And many, many more people in the organization should be able to get insights from the data. So we’re very excited about that.
Democratizing AI is different. Democratizing AI means practitioners … should be able to easily create AI models that understand your data and your organization. … We want you to be able to ask, ‘How’s the business doing with its FY [fiscal year] goals? … Business in your particular company means certain KPIs [key performance indicators] that are most important in your organization.
The definition of those, it should understand those, and then we want it to give you back authoritative answers that are certified and that are correct and that don’t have any hallucinations. … That’s what data intelligence is for us. … That’s what the whole company is working on. … When it comes to democratizing AI, that’s where our whole generative AI stack comes in. … We basically have … serverless GPUs around the world.
And we just enable you to very easily, seamlessly, in a UI, be able to build your own AI on your custom data and productionize it in about minutes. … We’re paying a lot for GPUs today, my CFO [David Conte] reminds me every week.
And that’s where you can run these—you can run a vector source database there. And then we have validation … how do you actually know your AI is doing well in production? And then … how do you govern it? How do we actually secure it? How do you make sure to track it … track the tokens? Make sure it’s not doing something that we don’t want it to do.
Making Databricks Serverless
All of Databricks now is available in serverless [starting July 1]. … whether it’s our notebooks or whether it’s our Spark clusters, whether it’s workflows, job processing, all the different aspects.
Databricks so far, only a few parts were serverless. Now you get all of it in serverless fashion. This is a project that has involved hundreds and hundreds of engineers for over two years. It’s been a long project internally. … There are no more clusters.
Everything just works super, super fast. … Today, you’re paying us for idle time if you’re not using serverless. Actually you’re paying the cloud vendors … and then you are paying us in addition to that, for idle time.
With serverless, you’re just paying for what you’re using. In fact, there is no cluster to set up for it to be idle … we’ll take care of all that for you under the hood.
And one thing that we’re excited about is we own all the machines now. It’s no longer this joint responsibility over machines that are running in your account and in our account. … You can really do the tracking and you can do the tagging and you can really use AI to predict where your costs are going in the serverless infrastructure.
And we’re also able to do security in a different way because we own all the machines to be able to lock it down in a different way. … All these knobs that we had before are gone.
Cluster tuning. … Spot instances. … Should it auto-scale? None of that is available anymore. … We just optimize it behind the scenes because it’s serverless. We’ll just run in the background optimization for your datasets to make it really fast and optimal using machine learning. … New products that we roll out next year … they’ll probably only be available in serverless.
Quest To A Standardized Format
We do have to agree on a standardized format. We put Delta Lake as one standard a bunch of years ago. It’s about 93 percent of all the data in Databricks. … It’s like way over 20 million machines every day crunched through that. … But there’s this other standard … Apache Iceberg, that emerged.
And it’s really not good for the customers in the world that all these organizations who wanted a [universal] format. … Imagine when there’s another plug that doesn’t work, that’s not good for anyone.
It is good for those that are being disrupted because they don’t want to get the data out of their proprietary data platforms. So our ulterior motive with this is to bring the formats closer so that we can get interoperability between these two formats, Apache Iceberg and Delta Lake.
We’re going to work with the communities to do that and want to, over the long term, make them 100 percent interoperable so that there is really no difference between them. … We do have UniForm, which is something that supports both formats. … Any one of our customers who uses UniForm will get the best of both worlds right away today.
And then for the next period of time, we’re going to bring the formats closer to each other. … In terms of exactly how are we going to move the two projects, that’s a very difficult, technical question that nobody on the planet right now has the answer to.
Ryan Blue, the original creator of Apache Iceberg [and CEO of Tabular] and Michael Armbrust, the original creator of Delta Lake [and Databricks distinguished software engineer] are going to work together and figure out exactly what those things look like.
Open-Source Contributor
We’ve committed … 12 million lines of code [to open-source projects] … I think it makes us, per engineer, the largest contributor of open-source software on the planet for an independent company today.
It used to be Red Hat. Now Red Hat is part of IBM. Per engineer, we contribute more to open source than any other company on the planet right now. And we work with these communities. … Any of these communities, if they don’t do well, like Apache Iceberg, Delta Lake … [Apache] Spark … If they don’t do well, it is not good for Databricks.
If any of these projects deteriorates and there is infighting and so on—which has happened with some open-source projects in the past—that would not be a good thing for Databricks.
It would not be good for the AI community, but it would really not be good for Databricks. It certainly wouldn’t be worth spending this much money for us to see that happen.
Snowflake Competition
As long as there are vendors, we are going to compete and there is going to be a fight between the different vendors. That is going to happen until the sun burns up. … I’m not too worried about [competition in] the catalog layer.
The catalog layer is very important. Our most important product at Databricks is Unity Catalog. … I’m not too worried about there being huge friction there. … Unity Catalog has supported both [common catalog standards] for over a year. And in fact, the Iceberg one, only Databricks and Tabular supported that API for almost a year.
None of the vendors out there that talk about this stuff has supported that. … What’s going to happen is maybe there are two implementations that are going to compete—the implementation we put out and maybe the implementation that [Snowflake] is going to put out in 90 days. Let the best one win.
But it doesn’t really matter to customers because if they’re buying services from us, they’re just using whatever we have in the cloud. … Only if they’re downloading these open source and running them, there are going to be two alternatives there.
Maybe there is going to be more. … The reason people love our catalog is that it’s also governance for AI. And what others have open-sourced and talked about they will open source is not for AI, just for tabular data. … We work with the community and those that have committers in the project. … If they happen to be employed by Snowflake … we’ll work with them as well. No problem.
Data Intelligence
The most important capability that our enterprises want in generative AI is how can they train the AI to use tools better instead of just coming up with words and hallucinating and making up stuff and being wrong.
Enterprises care about accuracy. So they want the AI to be able to, itself, go use different tools. Go use a calculator. Get the data from that vector database. … We also announced an agent SDK.
So building agents of LLMs, many agents that work together, is super important. That’s really the frontier of what our customers want to do today. … Governance of AI is super important.
In many large enterprises, it’s sort of like, ‘Oh my God, my employees are just randomly swiping credit cards. And they’re just sending our data to some random startup that’s doing GenAI. … Shut down all AI in the whole enterprise.’
That’s happening in many large organizations with hundreds of thousands of employees. So we announced … something that really helps those enterprises, which is you can now have all your calls to the AI go through an AI gateway that we also open-sourced … that way you can track who in your organization is calling which AI, where is your data going.
You can also put guardrails and filters. You can say, ‘PII data—personally identifiable information—should not go out.’ … You can also put rate limits to track your costs. And you can also set up security, access control, all that. … We have people that follow closely the regulations in different parts of the world. The EU [European Union] AI Act and so on … and making sure that we are making our customers as compliant as possible to that regulation. … For instance, the EU AI Act requires you to track exactly which model you’re using and track the provenance.
So if someone comes and asks, you should be able to show them. So we actually automatically are tracking that. … It’s really the stuff that enterprises need that we’re focused on. … Models that OpenAI and Anthropic are building, they are general intelligence. They can tell you about the Second World War. … We are focused on a different problem.
We’re not trying to answer those kinds of questions at Databricks. We are trying to help enterprises build AI that can answer questions on your private data in your organization. We call that data intelligence.
DBRX Updates
People have fine-tuned and created specialized AI custom models for enterprises, 200,000 over the last year on Databricks.
That’s not just [with Databricks’ LLM] DBRX. Some of it is DBRX. Some of it is [Facebook parent Meta’s] Llama. Some of it is other models.
I joked … that DBRX was the best model on the planet for two weeks until Llama came out. But actually … if you care a lot about speed, [DBRX] is still the smartest, fastest model.
If you just care about intelligence, pure intelligence, I would say Llama 3 is better. Also, if you care about the coding, it’s really, really good. So it really depends on the use cases.
So what we are seeing is people are combining many different AI models and building compound AI systems. … Verticalization is going to be important in AI. … And we’re actually partnering in each vertical. We have … Data Intelligence Platform for financial services. Data Intelligence Platform for media. … What we are seeing is that people want to mix and match.
In a complex system, you don’t want to just use one model, anyway. … The future is going to probably be more and more that way, is my guess. More and more specialization.
Because it turns out, when we trained DBRX … when we tried to make the model very good at some things it got worse at other things. DBRX was phenomenally good at English language. … But then when we started pushing it to become really good at programming, then its English actually deteriorated. … I don’t think you can have your cake and eat it too and be good at everything.
Open Source, Academia Key AI Communities
We are pro-open source. We think that it’s better that there’s a vibrant open-source community around these things. As we think with everything else … it’s better that it’s in the hands of open source than just one or two companies. … Research should be involved. … Academia does not have access to huge numbers of GPUs.
Portions of academia right now are pretty demoralized because they feel like, ‘Hey, we can’t actually do real research on generative AI properly because we don’t have access to the resources.’ … Open source is an important way for letting the researchers be part of this. … We need researchers to understand what AI models are doing and to be able to make them better and also deal with the risks that they may have. … If you want AI that’s really good at certain things, what’s the best way to get it to be really good at that particular thing?
Is it to train a completely new one from scratch? Is it to take an existing one and continue training it? Is it to take an existing one and just slightly tune it? Or are there other tricks?
I think this is the most important question, and nobody’s actually answered it. … Our research lab, we have about 50 researchers, should be focused just on this problem. … Our answer for our customers right now is we give them the whole framework so that they can explore and try out the different [options] for their particular problem.
We offer them the whole suite of things that they need from soup to nuts. They can try the different models.
Success With AI/BI, Warehousing Analytics
[Databricks’ new business intelligence product] AI/BI gives us the ability to get way broader audiences, like millions of people, that don’t know all the technical phrases … you just need to speak English. We’re very excited about that.
And we also built it such that you don’t have to have access to Databricks to use it in an organization. You don’t have to log into Databricks and have to give it permission. … Some of the performance measurements that we did compared to Snowflake, phenomenal performance, especially on BI.
The biggest breakthrough I think is how well we can do now on BI. Like if you plug in a BI tool to Databricks, we can give the best price/performance in the industry on that. … The data warehousing analytics product that we have is the fastest-growing product that we’ve ever launched.
And I think by any B2B standards worldwide, it’s one of the fastest-growing probably out there. … We launched it in December of 2020. And it has passed $400 million ARR [annual recurring revenue]. … So I think we’re doing very, very well. … We didn’t just say, ‘Hey, we’re going to build it a data warehouse and it’s good enough. And trust us it’s as good as what is out there.’
Then you can’t really win in that market. … You have to be 10 times better if you want to win. So when we launched our data warehouse, it was disruptive. … We disrupted the market by saying … it’s your own data, you own it yourself. … And you can do AI on top of it. So I think we have a disruptive approach to it. And I think that’s what actually enabled us to grow so fast.
Source link
lol
‘We both need to succeed together for Databricks to succeed,’ says Databricks co-founder and CEO Ali Ghodsi at the company’s Data+AI Summit. Databricks is “doubling down” on working with its systems integrator partners, with co-founder and CEO Ali Ghodsi viewing services partners as an important part of the vendor’s go-to-market and widespread adoption of artificial…
Recent Posts
- Arm To Seek Retrial In Qualcomm Case After Mixed Verdict
- Jury Sides With Qualcomm Over Arm In Case Related To Snapdragon X PC Chips
- Equinix Makes Dell AI Factory With Nvidia Available Through Partners
- AMD’s EPYC CPU Boss Seeks To Push Into SMB, Midmarket With Partners
- Fortinet Releases Security Updates for FortiManager | CISA