Phison President Promises AI Training, Tuning With A $50K Workstation
by nlqip
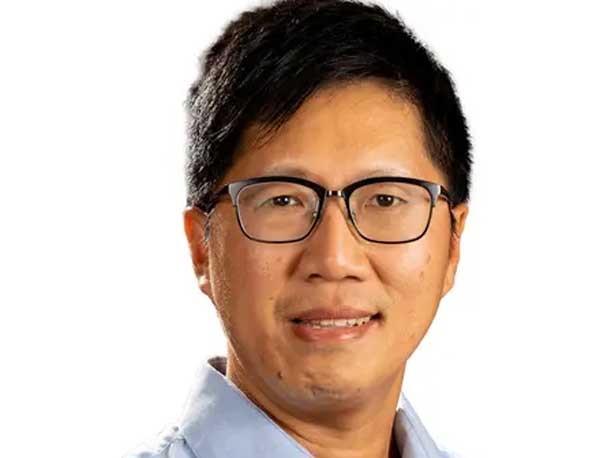
‘We’ve changed that $1 million or $1.5 million investment, the minimum requirement to have a fine-tuning machine to create ChatGPT, to $50,000. You no longer need three DGX GPUs anymore. You can do it with a single workstation with four workstation GPUs and with two of our aiDAPTIV+ SSDs that are treated as virtual memory for the GPU,’ says Phison General Manager and President Michael Wu.
Making AI Training, Tuning Available Outside The Big Enterprises
NAND controller and SSD developer Phison Electronics has flipped the switch on a new business focus with the recent unveiling of what it calls the first end-to-end on-premises GenAI system.
Dubbed the aiDAPTIVE+ Pro Suite, the new system is a software upgrade to the company’s aiDAPTIVCache AI100 SSD to provide everything needed to do on-premises data processing, training on very large language models, and add a chat interface to engage with the data, said Michael Wu, general manager and president of the Miaoli, Taiwan-based company with U.S. headquarters in San Jose, Calif.
With aiDAPTIVE+ Pro Suite, Phison is creating a memory expansion virtual port to the GPU VRAM, Wu told CRN.
[Related: Storage 100: The Digital Bridge Between The Cloud And On-Premises Worlds]
“People thought it’s not possible, but we did it,” he said. “AI requires super high endurance and performance. But most importantly, it’s not just the SSD. We actually created the driver that allows a host to look at a GPU card as if it has tons of memory for AI fine tuning and domain training.”
That approach is key to making AI available to a wider user community, given the fact that AI training and tuning previously was too expensive and hard to do for all but a few deep-pocketed companies, Wu said.
There is a lot going on behind Phison and aiDAPTIVE+. For the details, read CRN’s Q&A with Wu, which has been lightly edited for clarity.
Tell us about Phison.
In May we announced a brand-new enterprise lineup called Pascari. [Our first Pascari product is] our largest density, dual-port enterprise SSD at 64 terabytes using QLC flash. Large density, low-cost QLC is the number one darling these days in storage, so the timing is great. We also previewed 122.88-terabyte SSDs in the U.2 format. Now that is hard. Historically, it took quite some time to get from 16 terabytes to 32 terabytes, and all of a sudden people are crying for two more steps in a matter of six months. So we are definitely gearing up for that as well. We are going to bring it out sometime in Q4.
[Also,] we recently unveiled aiDAPTIV+, which allows us to create a memory expansion, a virtual port to the GPU VRAM. People thought it’s not possible, but we did it. AI requires super high endurance and performance. But most importantly, it’s not just the SSD. We actually created the driver that allows a host to look at a GPU card as if it has tons of memory for AI fine tuning and domain training. Everyone else in the AI world is talking about pre-training. ‘Oh, I don’t have enough H100 GPUs. They’re so expensive. Nvidia stock is going up. Meta is buying about 100,000 GPUs.’ All that is good. It’s building the infrastructure for the pre-training of ChatGPT and OpenAI and all that stuff.
How does aiDAPTIV+ fit in with what’s happening with AI?
People talk about AI PCs, AI phones, AI everything. Even AI monitors, but that’s a fake. All that is about AI inferencing. These are devices that have some inference capability that lets them take advantage of the pre-trained data. But an area that nobody is really looking at, but we think is very critical to build an infrastructure for, is domain training for businesses. You can’t expect businesses to be able to use ChatGPT with public knowledge immediately. People do it, but they take it home secretly, so the data is not going to the cloud. This is not just AI. It’s custom AI. You want it to be on-prem, but the cost of on-prem is so high. It took more than $1 million for my team to get the machine to train a Llama 3 70-billion parameter model. Just imagine that 70 billion parameters gives you all the information that you need. Just to compare, ChatGPT has 400 billion parameters because it has images and videos, everything. Businesses cannot live with small models. They have to fine tune their data to a big model so ChatGPT can be smart and have all your information. And that has to be on-prem.
We’ve changed that $1 million or $1.5 million investment, the minimum requirement to have a fine-tuning machine to create ChatGPT, to $50,000. You no longer need three DGX GPUs anymore. You can do it with a single workstation with four workstation GPUs and with two of our aiDAPTIV+ SSDs that are treated as virtual memory for the GPU. We’ve created a driver that allows the data to keep swapping back and forth.
Furthermore, when we demonstrated a 70-billion parameter machine at Nvidia’s GTC, people didn’t know how to use it. Nobody has an AI engineer. So we created a software tool called aiDAPTIV+ Pro Suite that lets you go from putting a PDF of your proprietary document to the system to fine tune the 70-billion parameter model to building a chatbot like ChatGPT. We are taking advantage of all the big investment that Meta has made on the open source Llama 3 to create a custom AI for you.
This is exciting because right now the whole world is telling you that HBM [high bandwidth memory] is a premium item. It’s a memory that goes on top of the GPU memory. For example, the Nvidia H100 GPU has 80 gigabytes of memory each. Each H100 could cost a quarter of a million dollars.
How do you use SSDs to act as virtual memory for GPUs?
The rule of thumb, and if you forget everything else from this call, you want to remember the ratio of 20. A 70-billion parameter model equals 70 gigabytes. 70 gigabytes times 20 equals 1.4 terabytes. So to train a 70-billion model, you need 1.4 terabyte of graphic card memory right now. This is the graphic card memory to be able to even finish a successful training. Today, you only have limited VRAM on a graphic card. The gating item, the bottleneck, of the training is the memory on the GPU. We are solving that problem with SSD. We call it the aiDAPTIVCache SSD. That’s the name of our SSD product line, adaptive cache. It’s not an SSD. It’s really used as a cache.
One last thing is, we think this technology, to be honest, is ahead of its time because businesses are not crying out loud for fine-turning business appliances. We call it a business appliance because we want it to be end-to-end. Customers want devices that, like a refrigerator, should just work.
One thing people don’t realize is that that’s assuming there’s no aiDAPTIV+, there’s no SSD offload. Today, without the SSD on the workstation, for example, you can only train 7 billion parameters. Even on an eight-DPU server, you could only train maybe 13 billion. Without our SSD technology, in three years you can improve your 7 billion to 13 billion on a workstation because of the HBM memory expansion. Even in 10 years, you still cannot touch a 70-billion parameter model, because memory scaling is just not fast enough. It’s much slower than model scaling. But even today, the biggest text-based model, 70 billion, you cannot tackle it without the SSD uploading. In 10 years, 2034, you can’t even do it. But we can do it today.
What’s the performance difference between aiDAPTIV+ and HBM?
If we compare the 70-billion parameter performance between a GPU only versus GPU plus aiDAPTIV+ on the workstation, you can’t compare because it doesn’t work. It takes four hours for us to train a 70-billion model, to fine-tune the 70-billion model, four hours for 10 million tokens. The token counts will impact the number of hours of training. So assuming 10 million tokens, it’s gonna take us 4.4 hours, and we plan to improve that to maybe three hours. On a 7-billion model with a GPU only, you could do that within an hour. So there is a difference. But our value proposition is that small and medium businesses, even if they train once a day, four hours is good enough. It’s nothing. You could do it overnight while your employees are at home sleeping.
The current GPU ecosystem is focused on the big players with effectively unlimited budgets. For them, speed is more important than cost, because they have a business model to monetize the result of their training. For most companies, it’s not nearly as clear cut, and so justifying millions for AI budget is hard.
There is a clear case to be made for larger models. They output better results and can compete 1:1 with OpenAI. But funding can be hard to generate. aiDAPTIV+ provides another option. If training or updating your model once a day works for your business, then you can access Llama 405B for the cost of a single workstation.
How about software?
Our technology has three parts. There is the SSD. And there is what we call aiDAPTIV+ Pro Suite middleware, which talks between the AI such as PyTorch and the graphic card with the SSD to manage the data traffic between the GPU and the SSD, and swap data. You can consider it as an SSD driver, but specialized for Phison. And the last part is the software interface called Pro Suite. Pro Suite is software that is included when you purchase the SSD. And so we become a software company, in that sense.
What sales channels does Phison use?
I’ll start from the Pascari SSD first. Phison has always been the machine behind the brand, doing ODM for our entire life. You cannot see a single Phison-branded SSD, whether client or industrial or whatsoever. Of our headcount, 75 percent is doing R&D. We are like the SSD team behind all the brands. This is the first time we are doing this for the enterprise, not the client. The go-to-market is just like Micron or Samsung. We’re gonna have the references. We have a brand. We have distributors, ASI and Ma Labs. We’re gonna have more.
For the aiDAPTIV+ side, AI is not like an SSD where you give it to someone and it will just work. So we are very focused on the direct design-in, meaning we’re working with the world’s top workstation makers right now. We want them to preinstall aiDAPTIV+ and get everything right. And a lot of them want to include the Pro Suite software.
The go-to-market strategy for aiDAPTIV+ is direct to the system integrators and workstation makers. We are also working with some channel partners because as a company, we don’t have a big direct database of all the end users that already have graphic cards or are already using or buying new systems. We have some channel partners that specialize in selling GPUs or helping end users build systems with GPUs. We’re leveraging them, providing training to tell them how to educate end customers. So we have added channel partners that add value to help promote this product right now. But I think the majority of sales will be more from new systems. I think the day of having this be as easy as an upgrade kit will still need some time.
Source link
lol
‘We’ve changed that $1 million or $1.5 million investment, the minimum requirement to have a fine-tuning machine to create ChatGPT, to $50,000. You no longer need three DGX GPUs anymore. You can do it with a single workstation with four workstation GPUs and with two of our aiDAPTIV+ SSDs that are treated as virtual memory…
Recent Posts
- Arm To Seek Retrial In Qualcomm Case After Mixed Verdict
- Jury Sides With Qualcomm Over Arm In Case Related To Snapdragon X PC Chips
- Equinix Makes Dell AI Factory With Nvidia Available Through Partners
- AMD’s EPYC CPU Boss Seeks To Push Into SMB, Midmarket With Partners
- Fortinet Releases Security Updates for FortiManager | CISA